Understanding Big Data Variety
Big data Variety refers to the rich array of different types of information collected and processed in a big data environment. It's one of the key characteristics of big data, also making up the 'V's of big data along with Volume, Velocity, and Veracity. Big data Variety includes structured, semi-structured, and unstructured data originating from multiple sources.
Define Variety in Big Data
Structurally, data can be divided into three types: structured, semi-structured, and unstructured. Understanding these classifications can greatly improve your grasp of big data Variety.- Structured Data: It is organized, tagged and easily searchable, often stored in traditional database systems. Examples include data in relational databases and spreadsheets.
- Semi-structured Data: This type of data contains some structured elements but lacks a rigid structure. Examples include XML files, email messages, and JSON data.
- Unstructured Data: This data lacks any particular form or structure and often comprises texts, videos, web pages, etc.
A practical visualization of big data Variety includes a social media platform like Twitter. It continually gathers structured data (e.g., user profiles, tweets, followers count), semi-structured data (e.g., hashtags, trending topics), and unstructured data (e.g., images, videos).
Characteristics of Big Data Variety
Big Data Variety exhibits a range of unique characteristics, including but not limited to:- Heterogeneity: The data is varied in nature, gathered from numerous sources.
- Anomalies: With varied data, there is an increased likelihood of inconsistencies, such as temporal and spatial anomalies.
- Complexity: Variety amplifies the complexity of data management, requiring sophisticated systems and algorithms.
- Incompatibilities: Different data types may lead to incompatible formats, representing a significant challenge for effective data integration.
Managing these characteristics requires specific techniques and tools. For example, capturing data from various sources and in different formats can benefit from an Extract, Transform, and Load (ETL) process.There's been significant evolution in the realm of data processing that leverages artificial intelligence and machine learning algorithms to handle the complexity of varied data. Tools like Apache Hadoop and Spark, NoSQL databases, and a rich ecosystem of data processing and analysis libraries in Python and R are prime examples of this continuing trend.
Examples of Big Data Variety
To better understand the concept of big data Variety, let's look at real-world examples.Structured data Credit card transaction data Semi-Structured data Email threads where important details are found in texts and attachments Unstructured data Social media posts containing texts, images, videos, locations, emojis, etc.
From these examples, you'll start to see how big data Variety incorporates information from diverse realms and formats. Its robust understanding and management are integral to unlocking the potential of big data.Exploring Variety and Variability in Big Data
In the realm of big data, your encounters span beyond mere volume or speed. There’s a significant interplay between Variety and Variability, two key 'V's characterising the complex big data landscape. While these terms sound similar, they highlight separate yet integral aspects of big data.
Differentiating Big Data Variety and Variability
Many might wonder about the difference between the two terms, considering they're often used interchangeably. Decoding their meanings can refine your understanding of big data complexities.Big Data Variety, as we've already discussed, refers to the different types of data we encounter, including structured, semi-structured, and unstructured data. It delineates the diverse sources and formats of the data being processed.
- Variety relates to diverse types of data - structured, semi-structured, unstructured.
- Variability implies changes or inconsistencies in data patterns over time.
- While Variety presents a challenge in terms of data processing and integration, Variability is about stability and predictive accuracy.
- Variety is tackled through robust data management systems while Variability requires potent predictive analytics tools and statistical modelling.
With high variability, data standardisation becomes a key challenge. Time series analysis, variance testing, anomaly detection, and other advanced predictive analytics and statistical approaches are often employed to curb the impact of high data variability. Additionally, sophisticated data mining algorithms can assist in detecting irregular patterns and adjusting predictive models accordingly. Importantly, the relationship between Variety and Variability in big data isn't isolated. With increased data diversity, there's a higher chance of finding variability within the data sets.The harmonisation of Variety and Variability in big data analysis serves as an underpinning for many real-world applications. For instance, in predicting stock market trends, data scientists rely on diverse data types (Variety) and consider changes over time (Variability) to construct more accurate predictive models.
Example of Difference Between Variety and Variability in Big Data
To bring these concepts closer to reality, it helps to examine real-world instances that underscore their distinctions and interactions. Consider the social media sphere, a fertile ground for big data generation. Here, big data Variety is encountered in different types of content users generate and interact with - textual posts, images, reactions, comments, etc.Big Data Variety User profiles, posts, comments, reactions Big Data Variability Varying user activity levels, temporal changes in interaction patterns
The Variability in this context could be in the form of fluctuating interaction rates - like the rate of comments on a provocative news post might see a sudden surge and die down after a while. Or, user activity patterns may display regular cycles - more activity during day hours as compared to nights, for instance.Another example might be an online retailer. The big data Variety they encounter is vast - user data, transaction data, website logs, customer feedback, and more. Variability manifests in the changes seen during festive sales when the traffic surges, transaction volumes rise, and customer queries increase.
Data Types in Big Data Analytics Variety
Unearthing the dynamism of big data Analytics Variety involves deciphering the multitude of data types. Big data analytics encompass a broad spectrum, existing across structured, semi-structured, and unstructured data repositories. Each data type presents unique opportunities and challenges. As such, understanding them holds the key to open up deeper, more meaningful explorations and insights.Identifying Data Types of Big Data Analytics Variety
Let's delve deeper into distinguishing among the three broad categories: structured, semi-structured, and unstructured data.
Structured Data: This data type encapsulates information with a high degree of organisation. It follows a clear, predefined model with identifiable patterns, allowing easy storage in relational databases and spreadsheets. In the world of big data, structured data inputs may include customer information, transaction data, or sensor data, to name a few. Structured data is highly amenable to queries, search, and processing because of its rigid structure. This inherent advantage makes it a popular choice for traditional data analytics tasks.
Semi-structured Data: A hybrid between structured and unstructured data, semi-structured data possesses some organised attributes but lacks a strict formal structure. It may include meta-tags, markers, or other labels that create an element of structure within the data. XML files and JSON data are typical examples of semi-structured data. Expressing semi-structured data in tabular form may not be very straightforward, but the partial structure aids in querying and analysis tasks.
Unstructured Data: Unstructured data includes data that does not conform to a specific format or model. This form of data is text-heavy but may contain data such as dates, numbers, and facts as well. Examples of unstructured data range from social media posts, video content, audio files to complex scientific data like weather patterns or astronomical observations. The key challenge with unstructured data is that it cannot be directly queried or processed and necessitates sophisticated analytical algorithms or human intervention for meaning extraction.
As you can see, each data type offers its own set of possibilities and hurdles. High-volume, high-velocity structured data might allow for real-time analytics, but only when good database designs are implemented. Semi-structured data dumps offer deep insights; however, they need effective parsing algorithms. Similarly, unstructured data contains rich and detailed information, but it requires sophisticated techniques, like machine learning or natural language processing, to unlock its value.Examples of Data Types in Big Data Analytics Variety
To solidify your understanding, let's examine specific instances that exemplify these data types. For instance, consider a large online retailer. They handle a blend of these data types daily:Structured Data Customer database containing information like id, name, contact details, purchase history Semi-Structured Data Email communications with customers containing structured fields (e.g., subject, date, recipient) and unstructured content (e.g., email body) Unstructured Data Customer reviews on products which largely consist of freeform text, but may also contain structured elements such as ratings
Or, suppose you're looking at a healthcare setup. The data here is a rich mix of structured records (like patient IDs, appointment schedules, prescription details), semi-structured content (like medical transcription records), and unstructured information (like patient notes or imaging data).
In these illustrations, note how different data types co-exist, capturing diverse yet complementary aspects of the business. Navigating these data types and understanding their interplay is crucial to maximise insights derived from analytics. Initial efforts may seem daunting, given the sheer scale of data. But remember, every data point embodies a story waiting to be discovered, and all combined, they provide a panoramic view of your function, be it retail, healthcare or any other sector.
Big Data Variety - Key takeaways
Big Data Variety refers to the different types of data collected and processed in a big data environment. It includes structured, semi-structured, and unstructured data.
Three main types of data in Big Data Variety are:
- Structured Data: Organized, tagged, and easily searchable data. e.g. data in relational databases and spreadsheets.
- Semi-structured Data: Contains structured elements but lacks a rigid structure. e.g. XML files, email messages, and JSON data.
- Unstructured Data: Lacks specific form or structure and often comprises texts, videos, web pages, etc.
- Big Data Variety is characterized by heterogeneity, anomalies, complexity, and incompatibilities.
- Big Data Variety and Variability are two different aspects of big data management. Variety refers to different types of data while Variability addresses the inconsistencies in data patterns.
- High data variability can be managed using time series analysis, variance testing, anomaly detection, and other predictive analytics and statistical approaches.
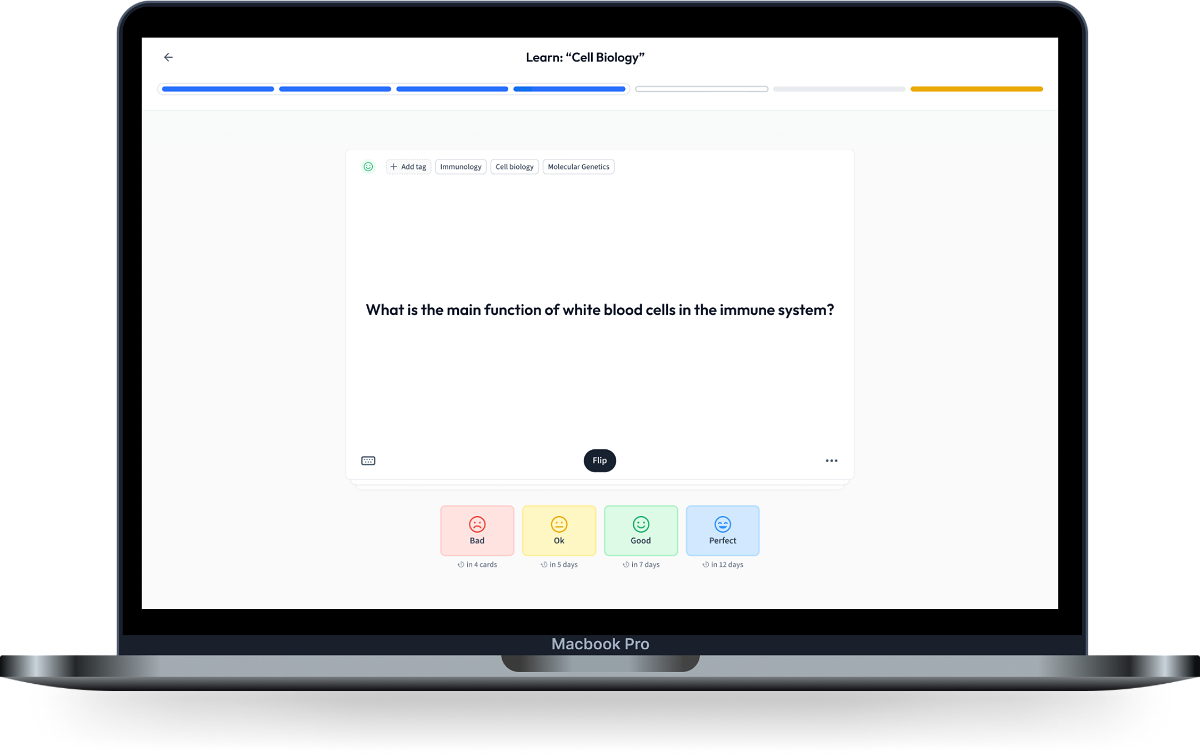
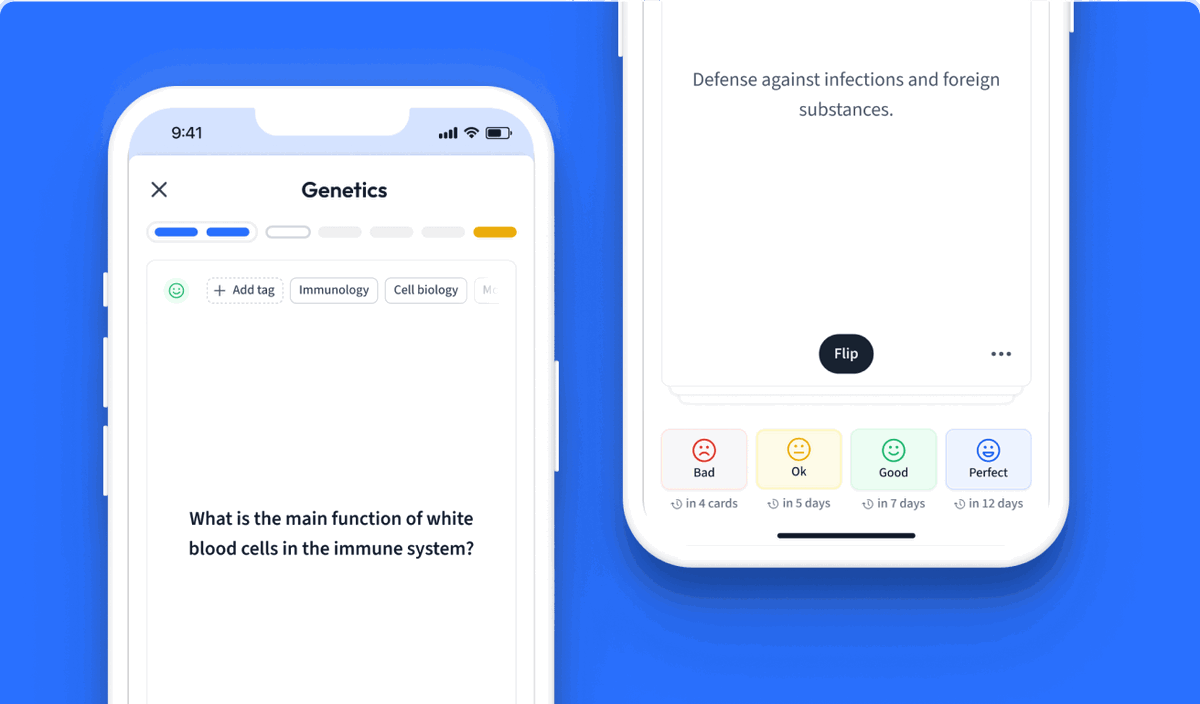
Learn with 15 Big Data Variety flashcards in the free StudySmarter app
We have 14,000 flashcards about Dynamic Landscapes.
Already have an account? Log in
Frequently Asked Questions about Big Data Variety
What is variety in big data?
What does variety in big data dimension means?
What is true about variety in big data?
What is the purpose of variety in big data?
What is variety characteristic of big data about?
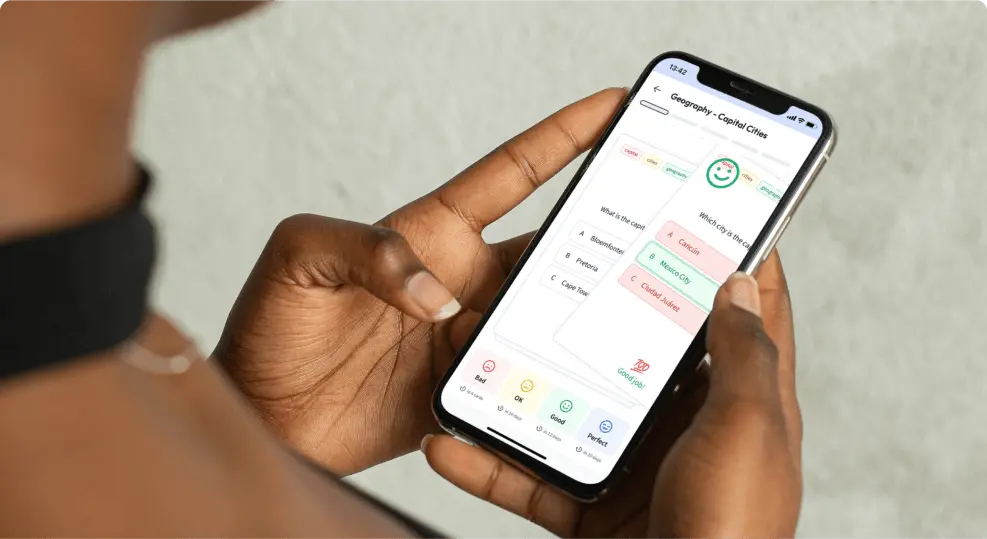
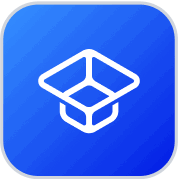
About StudySmarter
StudySmarter is a globally recognized educational technology company, offering a holistic learning platform designed for students of all ages and educational levels. Our platform provides learning support for a wide range of subjects, including STEM, Social Sciences, and Languages and also helps students to successfully master various tests and exams worldwide, such as GCSE, A Level, SAT, ACT, Abitur, and more. We offer an extensive library of learning materials, including interactive flashcards, comprehensive textbook solutions, and detailed explanations. The cutting-edge technology and tools we provide help students create their own learning materials. StudySmarter’s content is not only expert-verified but also regularly updated to ensure accuracy and relevance.
Learn more