Understanding the Two Way ANOVA
In the field of engineering and science, statistical analysis is a vital tool. One of the methods used extensively is Analysis of Variance or ANOVA. The Two Way ANOVA is a more sophisticated variant of this method.Decoding the Two Way ANOVA Meaning
Two Way ANOVA, also known as factorial ANOVA, looks at how two independent variables impact a dependent variable.Two independent variables: These are variables which you manipulate or control during your experiment or study.
Dependent variable: This is the outcome you measure based on the changes you make to your independent variables.
import statsmodels.api as sm from statsmodels.formula.api import ols model = ols('corrosion ~ temperature + humidity', data=yourdata).fit() anova_table = sm.stats.anova_lm(model, typ=2) print(anova_table)
Differences between One Way and Two Way ANOVA
While a One Way ANOVA focuses on the effects of a single independent variable, a Two Way ANOVA addresses multiple independent variables. - One Way ANOVA: \(F = \frac{{MS_{between}}}{{MS_{within}}}\) - Two Way ANOVA: \(F = \frac{{MS_{AB}}}{{MS_{within}}}\) Key differences:- Effect on dependent variables: One Way ANOVA only analyses the effect of one factor, whereas Two Way ANOVA checks the effect of two factors simultaneously.
- Interaction effects: The Two Way ANOVA also considers the interaction effects between the two factors. This is something the One Way ANOVA can not do.
Deep Dive into Two Way ANOVA Properties
The Two Way ANOVA is a statistical test that lets you examine multiple factors at the same time.Underlying Assumptions of Two Way ANOVA
The Two way ANOVA, like any other statistical test, operates under certain assumptions:- Normality: The responses for each combination of levels of the factors follow a normal distribution.
- Independence: The observations for each combination of the levels of the factors are independent of each other.
- Equality of variance: The variances of the responses for each combination of the levels of the factors are all equal. This is also known as the assumption of homoscedasticity.
Variability Analysis in Two Way ANOVA Properties
The essence of Two Way ANOVA is the decomposition of overall variability into separate components.Total variability: It is the variability in the measured data (say the corrosion rate of a metal). It is represented by SST (Sum of Squares Total).
Say in an engineering experiment on corrosion, the SST is 600 units of which the temperature (SSA) is contributing 100 units, the humidity (SSB) 150 units, and their interaction (SSAB) another 50 units. The remaining variability (SSE) would be 300 units.
Practical Applications of Two Way ANOVA
Two way Analysis of Variance (ANOVA) is an extremely useful statistical tool for engineers and scientists as it helps analyse the effects of two independent variables on a response variable. It goes a step beyond the One Way ANOVA by considering both the individual effects of two factors, as well as their interaction.Two Way ANOVA Applications in Engineering Mathematics
In the realm of engineering, Two Way ANOVA finds a myriad of applications. Key among these is in experiment design and quality control. In experimental design, it aids engineers in understanding how different factors, and their interactions, influence the outcome of an experiment. Notably, it is used to:- Optimize processes: Two Way ANOVA helps determine the levels of independent variables that will lead to the most desirable outcome for the response variable. This is advantageous in scenarios such as industrial manufacturing where process optimization saves resources and time.
- Improve product quality: In quality control, it is used to ascertain how different factors and their interaction affect the quality of products. Here, the factors could range from raw materials to production methods.
Real Life Examples of Two Way ANOVA Applications
To illustrate this, let's consider a real-world engineering experiment. Suppose an engineer wants to understand how the hardness and temperature of a metal affect its corrosion. Two Way ANOVA is used to look at four groups: high hardness and high temperature, high hardness and low temperature, low hardness and high temperature, and low hardness and low temperature. Sample Python code:import statsmodels.api as sm from statsmodels.formula.api import ols model = ols('corrosion ~ temp + hardness + temp:hardness', data=metalexperiment).fit() anova_table = sm.stats.anova_lm(model, typ=2) print(anova_table)There's ample evidence of such statistical analysis in scientific literature. For instance, a published paper "Effect of Heat Treatment on Some Mechanical Properties of 7075 Aluminium Alloy" uses Two Way ANOVA to understand the effect of heat and pressure to optimize the alloy's mechanical properties.
Interpreting Results from Two Way ANOVA Applications
Interpretation of the analysis begins with examining the resultant ANOVA table. A typical ANOVA table would look something like this:Source | SS | df | MS | F | P-value |
Temperature | SSA | DF_A | MSA | F_A | P_A |
Hardness | SSB | DF_B | MSB | F_B | P_B |
Temperature:Hardness | SSAB | DF_AB | MSAB | F_AB | P_AB |
Error | SSE | DF_E | MSE | ||
Total | SST | DF_T | MST |
The Mathematical Dimensions of Two Way ANOVA
Embarking into the mathematical dimensions of Two Way ANOVA, we delve into its edifice and foundation. The crux lies in understanding its grand formula and learning from veritable examples.Understanding the Two Way ANOVA Formula
The Two Way ANOVA springs from the aim to understand how two factors affect an outcome and if there's an interaction between them. In this quest, it creates a blueprint called the Two Way ANOVA formula which defines how the distribution of data can be analysed. The run-of-the-mill Two Way ANOVA model is given by: \[ Y_{ijk} = \mu + A_i + B_j + (AB)_{ij} + \epsilon_{ijk} \] Where: - \(Y_{ijk}\) is the kth observation on the ith level of factor A and the jth level of factor B. - \(\mu\) is the overall mean. - \(A_i\) is the effect of the ith level of factor A. - \(B_j\) is the effect of the jth level of factor B. - \((AB)_{ij}\) is the interaction effect between the ith level of factor A and the jth level of factor B. - \(\epsilon_{ijk}\) is the random error component. Now, we unmask the F-ratios. An F-ratio is the ratio of variation between group means (MSA, MSB, MSAB) to the variation within groups (MSE). The F-ratios for the main effects and interaction in a Two Way ANOVA can be expressed as: \[ F_A = \frac{MSA}{MSE}\] \[ F_B = \frac{MSB}{MSE}\] \[ F_{AB} = \frac{MSAB}{MSE}\] If the computed F-ratios for A, B or AB are large, it suggests that there is a considerable variation explained by the respective factor or interaction. These F-ratios, once calculated, are compared against a critical F-value in the F-distribution table. P-values are then found, and if they are less than the conventional 0.05 level, it rejects the null hypothesis that there's no difference in means due to the respective factor or interaction.Dissecting the Components of the Two Way ANOVA Formula
Breaking down the formula, it swims down to various interrelated elements: the overall mean (\(\mu\)), the main effects (A and B), the interaction effect (AB) and the error (\(\epsilon\)). The main effects (A and B) are the influences that the respective factors have on the response variable, preferably taken individually. In a real-world example, say in an experiment testing a metal's stress-corrosion cracking time, the factors might be temperature and oxygen concentration. The effects A and B would quantify the individual effects of temperature and oxygen concentration on the cracking time. The interaction effect (AB) examines if there's an interaction between the two factors. Does changing the temperature and oxygen concentration at the same time have a different effect on the cracking time than just changing them individually? To underline this, here's a line of Python code that models the Two Way ANOVA, which includes the interaction term as well:meta = ols('cracking_time ~ temperature + concentration + temperature:concentration', data=metaexperiment).fit()The error (\(\epsilon\)) is the random component which accounts for the variability not explained by the factors A and B or their interaction. Taking the quintessential example, the test statistic (F_A) to see if temperature makes a difference is computed as: \[ F_A = \frac{MS_{temperature}}{MS_{error}}\] Likewise for factor B (oxygen concentration) and the interaction AB. Understanding this dissection, it's now elementary to look at some examples.
Exploring Two Way ANOVA Examples and Solutions
An engineer studying how the pipeline material and pressure affect the fluid flow rate would use a Two Way ANOVA. The two materials are Steel and Bronze (Factor A), the three pressure levels are Low, Medium, and High (Factor B). The engineer collects flow measures on different pipes made of these materials at various pressures. A Two Way ANOVA can verify if material, pressure or their interaction enhances the fluid flow. Computational tools like Python can be used to perform the Two Way ANOVA:pipe = ols('fluid_flow ~ material + pressure + material:pressure', data=pipeexperiment).fit() anova_table = sm.stats.anova_lm(pipe, typ=2) print(anova_table)The Python output gives the sum of squares (SS), degrees of freedom (df), mean square (MS), F statistic (F), and the P-value for each factor and the interaction. The crux lies in deciphering the P-values. For instance, a P-value of 0.032 for material means the null hypothesis, that there's no differential effect of material on flow rate, is rejected at a 0.05 significance level. Subsequently, material does make a significant difference to the flow rate. This journey into the mathematical dimensions of Two Way ANOVA is illustrative and rewarding. It underlies how intricate and yet approachable Two Way ANOVA—a grand tool in the engineering realm and beyond—truly is.
The Two Way ANOVA Test
The Two Way ANOVA, short for Analysis of Variance, is a robust statistical test that allows you to compare the means of multiple groups influenced by two distinct factors. It provides a comprehensive report by taking into account the individual effects of each factor and their interactions as well.How to Conduct a Two Way ANOVA Test?
A Two Way ANOVA Test, while complicated at first glance, can be conducted in a step-by-step manner. The key to a successful ANOVA test is to maintain a systematic and orderly approach. Ensure to be familiarised with the statistical software you'll employ to conduct the test, prepare the dataset thoroughly, and interpret the results meticulously. The principal steps in conducting a Two Way ANOVA are as follows:- Formulate a clear hypothesis.
- Collect your data and ascertain that it meets ANOVA assumptions - normality, homogeneity of variances, and independence of observations.
- Enter the data into the statistical software and perform the test.
- Interpret the output, focusing on the F-statistics and the associated p-values.
- Reach conclusions based on the results and support them with statistical evidence.
Step by Step Guide to Performing a Two Way ANOVA Test
The Two Way ANOVA begins with a clear statement of the problem and the formulation of null and alternative hypotheses. For a Two Way ANOVA analysing the factors A and B, these hypotheses might be:\[ H_0 : \mu_{A1} = \mu_{A2} = ... = \mu_{Am}\] \[ H_a : \text{At least one } \mu_{Ai} \text{ differs}\]
model = ols('Outcome ~ C(FactorA) + C(FactorB) + C(FactorA):C(FactorB)', data=mydata).fit() anova_table = sm.stats.anova_lm(model, typ=2)This snippet fits the model, performs the Two Way ANOVA and saves the table of results. Finally, interpret the results with attention to the F-statistics and the corresponding p-values. The p-value indicates the probability that you'd see a result as extreme or more extreme given if the null hypothesis was true. You have to make your decision for each factor and their interaction based on these p-values. If the p-value is lesser than your significance level (usually 0.05), you reject the null hypothesis for that factor.
Interpreting Results of Two Way ANOVA Test
Interpreting the results hinges largely on understanding the output table which commonly contains the following components: the source of variation (Factor A, Factor B, Interaction AB, and Error), sums of squares (SS), degrees of freedom (df), mean squares (MS), F value, and the p-value. The crux of interpretation relies on the F-values and the associated p-values. As a thumb rule, if the p-value is less than 0.05 (or your chosen significance level), it indicates a statistically significant effect. Further, if the interaction term is significant, it means that the effect of one factor depends on the level of the other factor. Don't forget to check the residual plots as they provide valuable diagnostic information. They should ideally show a random scattering of points which would indicate constant variance and independent errors.Common Mistakes in Two Way ANOVA Test Analysis
The path to conducting and interpreting a Two Way ANOVA test is laden with potential pitfalls. Beware of these commonly encountered mistakes:
- Misunderstanding interaction: Many people misconstrue significant main effects to mean that there's no interaction. It's crucial to remember that the main effect and interaction are distinctly different and should be interpreted separately.
- Ignoring assumptions: ANOVA has certain assumptions. If these assumptions are violated and the problem is not rectified, the results from the ANOVA could be misleading.
- Overlooking post-hoc tests: If you find a significant main effect, the story doesn't end there. You have to conduct post-hoc tests to understand where the differences lie.
- Confusing statistical significance with practical importance: Just because a result is statistically significant does not mean it's practically relevant. Remember to consider real-world implications and utility.
Two Way ANOVA - Key takeaways
- Two Way ANOVA is a statistical test that allows examination of multiple factors at the same time.
- The underlying assumptions for Two Way ANOVA include normality, independence and equality of variance.
- The total variability in Two Way ANOVA is represented by SST (Sum of Squares Total) and can be decomposed into SSA (factor A), SSB (factor B), SSAB (interaction between A and B), and SSE (remaining or residual variability).
- Two Way ANOVA is commonly used in engineering for experiment design and quality control, to optimize processes and improve product quality.
- The Two Way ANOVA test allows the comparison of the means of multiple groups influenced by two distinct factors, focusing on the individual effects of each factor and their interactions.
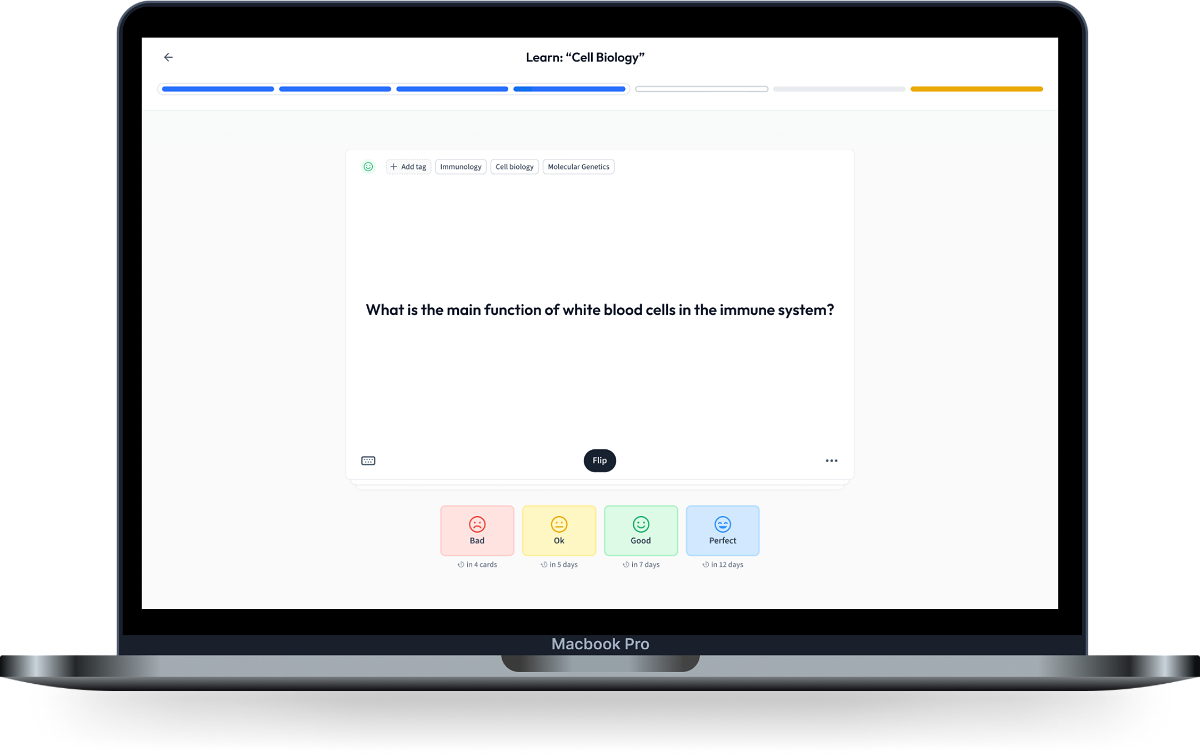
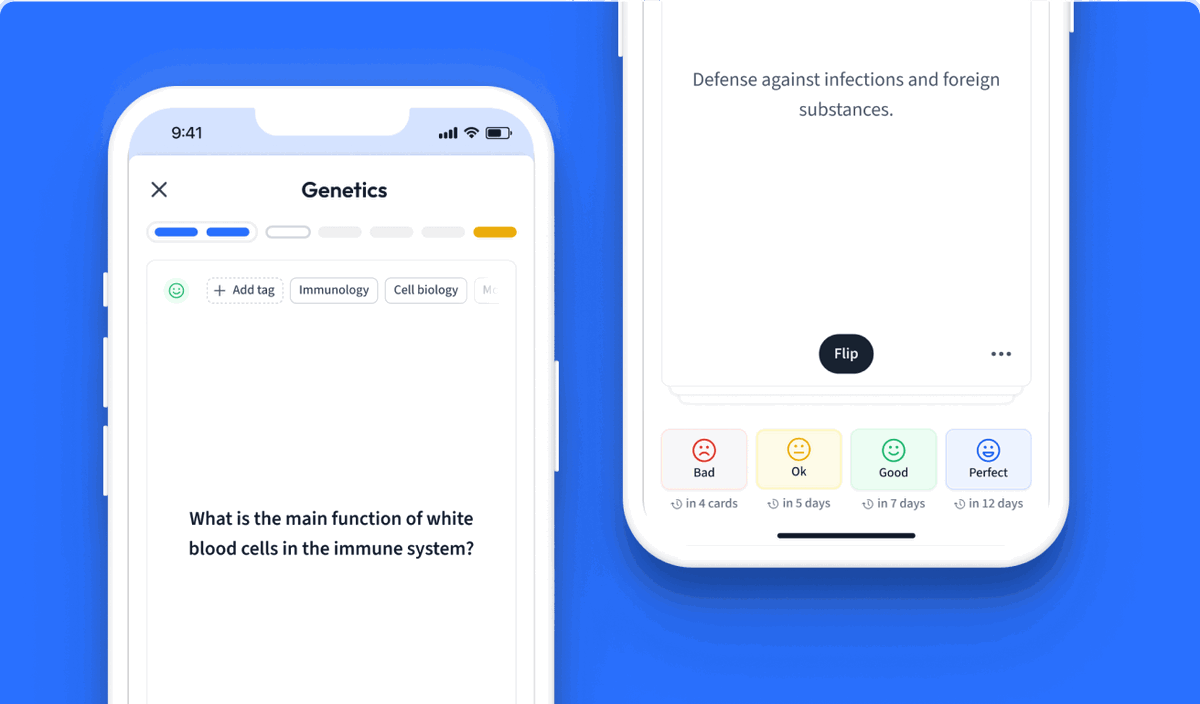
Learn with 12 Two Way ANOVA flashcards in the free StudySmarter app
We have 14,000 flashcards about Dynamic Landscapes.
Already have an account? Log in
Frequently Asked Questions about Two Way ANOVA
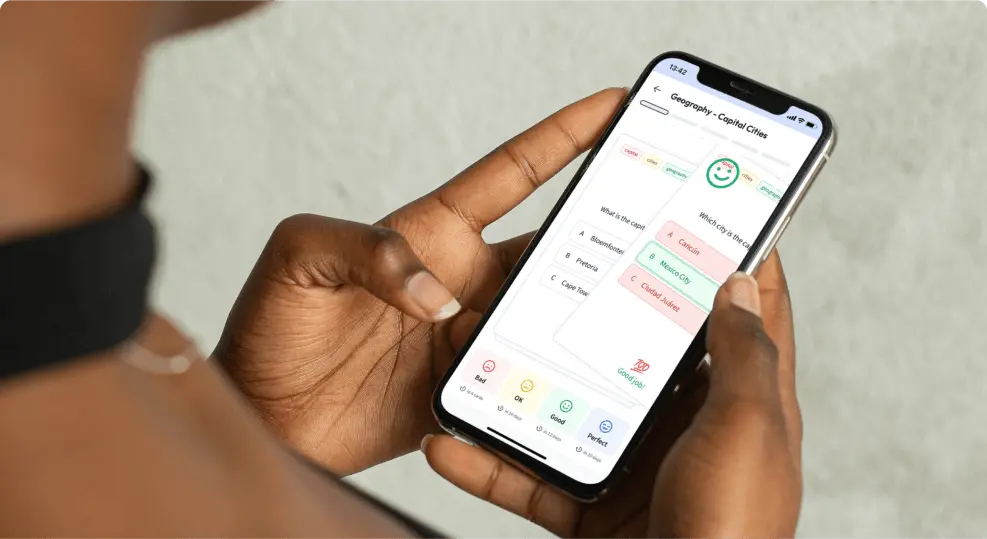
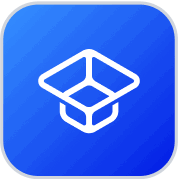
About StudySmarter
StudySmarter is a globally recognized educational technology company, offering a holistic learning platform designed for students of all ages and educational levels. Our platform provides learning support for a wide range of subjects, including STEM, Social Sciences, and Languages and also helps students to successfully master various tests and exams worldwide, such as GCSE, A Level, SAT, ACT, Abitur, and more. We offer an extensive library of learning materials, including interactive flashcards, comprehensive textbook solutions, and detailed explanations. The cutting-edge technology and tools we provide help students create their own learning materials. StudySmarter’s content is not only expert-verified but also regularly updated to ensure accuracy and relevance.
Learn more