Understanding Analytical Techniques in English Language
Analytical techniques are crucial for better comprehension and interpretation of texts in the English language. They help in identifying different linguistic elements and the relationships between them, leading to a deeper understanding of the message the text attempts to convey.Importance of Analytical Techniques in Linguistics
English language learners and literary enthusiasts often find themselves challenged by complex texts and the hidden meanings within them. The importance of analytical techniques in linguistics lies in their ability to facilitate the interpretation and understanding of texts.Analytical techniques, as employed in linguistics, are methodologies or strategies used to examine and interpret linguistic data, more specifically, texts.
- Investigate the structure and organization of linguistic data
- Identify patterns and relationships between different elements
- Reveal hidden meanings and symbolic references
- Evaluate the effectiveness of communication strategies
- Improve your comprehension, reading, and writing skills
Examples of analytical techniques in linguistics:
- Content analysis: focuses on analysing the content of a text in terms of recurring themes and patterns
- Discourse analysis: examines the ways in which language constructs meaning in different types of texts
- Stylistic analysis: investigates the use of specific linguistic features, such as syntax, lexicon, and rhetorical devices, and their impact on meaning
The Role of Text Analytics Techniques
Text analytics techniques play an integral role in deriving valuable insights and understanding from a text. In the context of the English language, these methods help you to explore different aspects of written communication more effectively.Text analytics techniques are used in various fields, such as digital humanities, marketing, and social research, to extract information and insights from vast amounts of textual data.
Type | Focus |
Lexical | Analysis of words, phrases, and their relationships |
Grammatical | Examination of the structure and syntax of sentences |
- Text classification: categorizing texts based on their content, such as topic or sentiment
- Named entity recognition: identifying and categorizing named entities, such as names of people, organizations, and locations within a text
- Part-of-speech tagging: assigning grammatical roles to words, like nouns, verbs, and adjectives
- Dependency parsing: determining the relationships between words in a sentence and their syntactic functions
Imagine you're working on a research project concerning the themes of climate change in newspaper articles. By employing text analytics techniques, you can classify articles based on their sentiment towards the issue, extract relevant entities like organizations and events, and discern patterns in the way the subject is discussed.
Different Types of Analytical Techniques
In the realm of English language analysis, we find a plethora of analytical techniques designed to extract meaning and insights from texts. These techniques cover a wide scope, ranging from predictive analytics to structured and advanced analytics approaches. Each type serves a specific purpose within the linguistic analysis framework and has unique benefits and applications.Predictive Analytics Techniques in English Language
Predictive analytics techniques focus on using historical data to make predictions and identify trends in language patterns. By using algorithms, statistical models, and computational methods, these techniques allow you to foresee particular linguistic characteristics, themes, and potential meanings in texts. Some of the widely-used predictive analytics techniques include:- Regression analysis: a statistical method that examines the relationships between variables and predicts their future values
- Time series analysis: a technique used to analyse data collected over a period of time to identify underlying patterns and trends
- Text mining: the process of extracting useful information from vast volumes of unstructured text data
- Machine learning: a subset of artificial intelligence that employs algorithms to automatically learn from data and improve prediction accuracy
In the context of the English language, you could use predictive analytics techniques to gain insights into evolving language patterns and trends over time. For example, by analysing historical data, you can predict which words or phrases are likely to decline in usage or popularity in the future.
Structured Analytic Techniques and Their Uses
Structured analytic techniques involve a systematic and organized approach to analysing texts in the English language. These methods primarily focus on breaking down the text into constituent parts and examining their relationships, emphasizing clarity and logic in the process of language analysis. Some advantages of structured analytic techniques include:- Reduced cognitive biases and errors in analysis
- Increased transparency and consistency in the analytical process
- Improved decision-making based on logically-structured insights
- Matrix analysis: organising information in a two-dimensional table to examine relationships and patterns
- Inductive and deductive logic: using general principles to make specific predictions (inductive) or using specific observations to draw general conclusions (deductive)
- Timeline analysis: arranging events and information in chronological order to understand the sequence and interactions between them
- Flowchart analysis: using a graphical representation to illustrate a process or a system of relationships
For instance, if you are analysing the structure of a novel, you can use a matrix to compare different characters based on their traits, actions, and motivations. This structured approach allows you to identify patterns, similarities, and differences across the text systematically.
Advanced Analytics Techniques for Language Analysis
Advanced analytics techniques, often supported by machine learning and artificial intelligence, enable deeper and more comprehensive analyses of the English language. These methods go beyond traditional approaches by incorporating innovative algorithms and computational models to analyse linguistic data more effectively. Some of these advanced techniques consist of:- Text clustering: grouping similar texts or passages based on their content, using unsupervised machine learning algorithms
- Sentiment analysis: using natural language processing algorithms to determine the sentiment or emotion expressed in a text
- Topic modelling: identifying and hierarchically classifying the topics discussed in a text or a set of documents
- Word embedding: converting words into numerical representations (vectors) that capture semantic meaning and can be used in machine learning models
Suppose you are analysing thousands of news articles on a particular subject, such as climate change. You can use advanced analytics techniques like topic modelling to automatically extract and categorise the most prominent themes in your dataset, allowing for a more efficient and comprehensive analysis.
In-depth Look at Descriptive and Prescriptive Analytics Techniques
When applied to the domain of English language analysis, descriptive and prescriptive analytics techniques offer unique approaches for understanding and enhancing language use. Each category of techniques dives into specific aspects of linguistic analysis, providing valuable insights and guidance for effective communication.
Descriptive Analytics Techniques for Language Understanding
Descriptive analytics techniques are designed to summarise and illustrate linguistic data, enabling you to identify patterns, connections, and trends in a text. By employing these methods, you can develop a comprehensive understanding of language use in various texts or situations, thereby strengthening your ability to interpret and assess written and spoken communication. Common descriptive analytics techniques for language understanding include:- Frequency analysis: examining the occurrences of words, phrases, or linguistic features in a text or corpus for identifying patterns and trends
- Collocation analysis: investigating the co-occurrence of words or phrases in a text to reveal underlying meanings and contextual relationships
- Concordancing: creating an alphabetical list of words or phrases from a text, along with their immediate context, for comparative analysis
- Word cloud generation: visually representing the frequency of words in a text, with more frequently-used words appearing larger in the graphic
For example, when exploring speeches made by political leaders, you can perform a frequency analysis to identify the most commonly used words and phrases, providing insights into the key issues and concerns discussed. Furthermore, a collocation analysis can reveal the connections between these words and phrases, shedding light on the overall message being conveyed.
Prescriptive Analytics Techniques for Linguistic Improvement
Prescriptive analytics techniques delve into the realm of providing actionable recommendations and guidance based on the analysis of linguistic data. These methods are especially valuable for identifying areas of improvement in written and spoken communication, as well as suggesting ways to enhance language use for more effective communication. The prescriptive analytics techniques focus on:- Error detection and correction: identifying grammatical, syntactical, and lexical errors in a text and offering suggestions for improvement
- Stylistic recommendations: providing suggestions for language use that align with specific genres, audiences, or purposes, such as academic writing or business communication
- Readability enhancement: evaluating the complexity and comprehensibility of a text and suggesting ways to improve its readability for the intended audience
- Text summarisation: extracting the main ideas and essential information from a text to create a concise summary, highlighting its most important aspects
Imagine working on a research paper in which you need to adhere to strict academic writing guidelines. By using prescriptive analytics techniques, you can identify and correct any language errors, ensure stylistic consistency, and enhance readability, resulting in a polished and high-quality document.
Exploring Modelling Techniques in Predictive Analytics
When it comes to analysing the English language through predictive analytics, numerous modelling techniques can be applied to uncover patterns, trends, and structure in texts. These techniques are particularly useful for enhancing the understanding of linguistic features and improving communication.
Types of Modelling Techniques for English Language Analysis
Various modelling techniques can be employed for English language analysis, each with its own strengths and applicability. These techniques draw on different fields such as statistics, machine learning, and natural language processing to build informative models. Some of the most prominent techniques include:
- N-gram models: capture the probability of a word or phrase occurring given the sequence of words that preceded it, where 'n' represents the number of words in the sequence. They are useful for studying the co-occurrence patterns between words and phrases.
- Hidden Markov models: a statistical model that captures the probability of transitioning between different states in a sequence. In language analysis, states are typically represented by words or linguistic features, and the model can assist in predicting the most likely sequence of words in a given context.
- Decision trees: a hierarchical model that makes predictions by branching out a series of decision rules. In the context of language analysis, decision trees can be employed to classify texts or linguistic features based on specific criteria.
- Neural networks: a type of machine learning model inspired by the architecture of the human brain. They are designed to recognise and learn complex patterns in data. In the English language, neural networks can be employed for tasks such as language translation, sentiment analysis, and error correction.
- Topic modelling: a group of unsupervised learning methods that focus on extracting the underlying structure and thematic patterns from a corpus of texts. Latent Dirichlet Allocation (LDA) is a popular topic modelling technique that identifies the distribution of topics across documents and the distribution of words within topics.
How to Apply Modelling Techniques in Linguistics
Applying modelling techniques in linguistics involves a carefully planned approach that depends on your analysis objectives and the nature of the data. The following steps outline a comprehensive process for applying these techniques to linguistic analysis:
- Data collection and preprocessing: Collecting a representative and diverse sample of texts or linguistic data for your analysis is imperative. This step involves data cleaning, such as removing irrelevant information, correcting errors, and normalising text to ensure consistent formatting.
- Feature extraction: Identifying relevant linguistic features (e.g. word frequency, sentence length, part-of-speech tags) from the text data is crucial for building an informative model. These selected features should align with the analysis objectives and capture essential aspects of the textual information.
- Model selection: Choose the most suitable modelling technique based on your analysis objectives, the nature of your data, and the complexity of the linguistic features. It is crucial to understand the strengths and limitations of each technique to make an informed decision.
- Model training: Train your selected model on a portion of your dataset. This process involves adjusting the model parameters to minimise the error between the model's predictions and the actual data. Supervised learning techniques, such as decision trees and neural networks, require labelled data for training.
- Model evaluation and validation: Assess the performance of your model by comparing its predictions to the actual data. This evaluation can be done using cross-validation techniques or by comparing the results to a predefined benchmark. It is crucial to ensure that the model is not overfitting, meaning it performs well only on the training data but poorly on unseen data.
- Model deployment and interpretation: Once your model is trained and validated, apply it to new data to uncover insights and make predictions relevant to your linguistic analysis objectives. Interpret the model's findings and discuss their implications, keeping in mind the limitations and possible biases of your chosen technique.
Enhancing Your Analytical Skills with Various Analytical Techniques
Developing your analytical skills in the English language is crucial to tackle complex texts, interpret diverse ideas and foster clearer communication. Employing various analytical techniques can aid in enhancing your ability to explore language patterns, themes and nuances more effectively.
Tips for Choosing the Right Analytical Technique
Selecting the most appropriate analytical technique for your linguistic task can significantly impact the depth and accuracy of your analysis. To make an informed decision, consider the following factors:- Analysis Objectives: Define your specific goals and requirements for the analysis, such as identifying language structure, uncovering hidden meanings, examining stylistic elements or predicting linguistic trends.
- Data Characteristics: Consider the nature of your textual data, including its size, format, complexity and the type of language features you wish to examine.
- Technique Strengths and Limitations: Understand the unique capabilities and restrictions of each analytical technique, as well as their applicability to your objectives and data characteristics.
- Computational Requirements: Evaluate the level of computational power and resources needed for implementing the chosen technique, based on your available tools and infrastructure.
Practical Application of Analytical Techniques in Education
The use of analytical techniques in education extends beyond academic studies of language and literature, with broader applications for developing students' linguistic and communication skills. Through incorporating these analytical methods into classroom activities and exercises, educators can facilitate better understanding and appreciation of language use, structure and complexity.Examples of practical applications of analytical techniques in education:
- Text Analysis: Encourage students to apply content analysis, discourse analysis and stylistic analysis to dissect various types of texts, fostering a deeper comprehension of language patterns, themes, and the impact of specific linguistic features.
- Group Discussions: Engage students in group discussions and debates, utilising analytical techniques like matrix analysis and flowchart analysis to help them organise their thoughts and arguments systematically.
- Writing Exercises: Incorporate prescriptive analytics techniques into writing exercises by providing students with feedback on their written work and guiding them towards improved language use, stylistic consistency, and readability.
- Research Projects: Encourage students to employ advanced analytics techniques, such as text clustering, sentiment analysis, and topic modelling, in their research projects to derive valuable insights from large datasets of textual information.
- Model Building: Introduce students to various modelling techniques in predictive analytics, such as N-gram models, decision trees, and neural networks, and allow them to explore their applicability in linguistic analysis tasks like language prediction, error correction, and sentiment analysis.
Analytical Techniques - Key takeaways
Analytical techniques play a significant role in understanding and enhancing the learning experience for English language students.
Predictive analytics techniques focus on using historical data to make predictions and identify trends in language patterns.
Structured analytic techniques involve a systematic and organized approach to analysing texts in the English language.
Descriptive and prescriptive analytics techniques aid in language understanding and improvement, respectively.
Various modelling techniques can be applied to uncover patterns, trends, and structure in texts to enhance the understanding of linguistic features and improve communication.
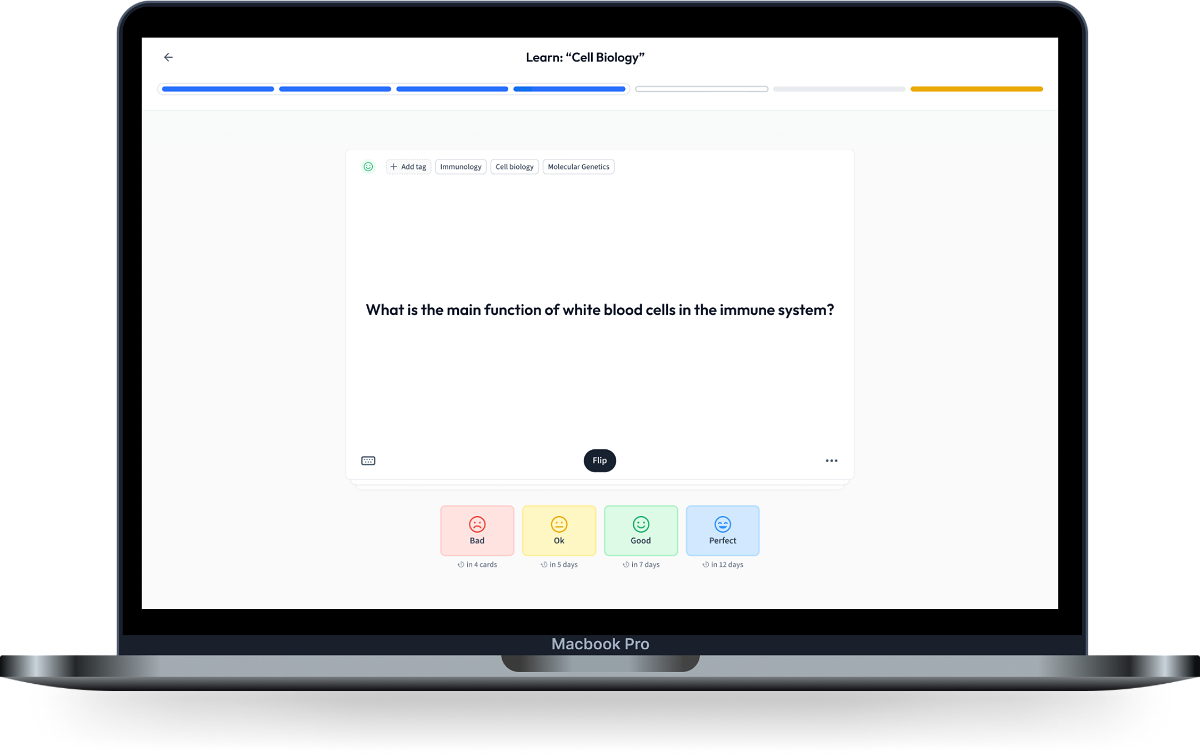
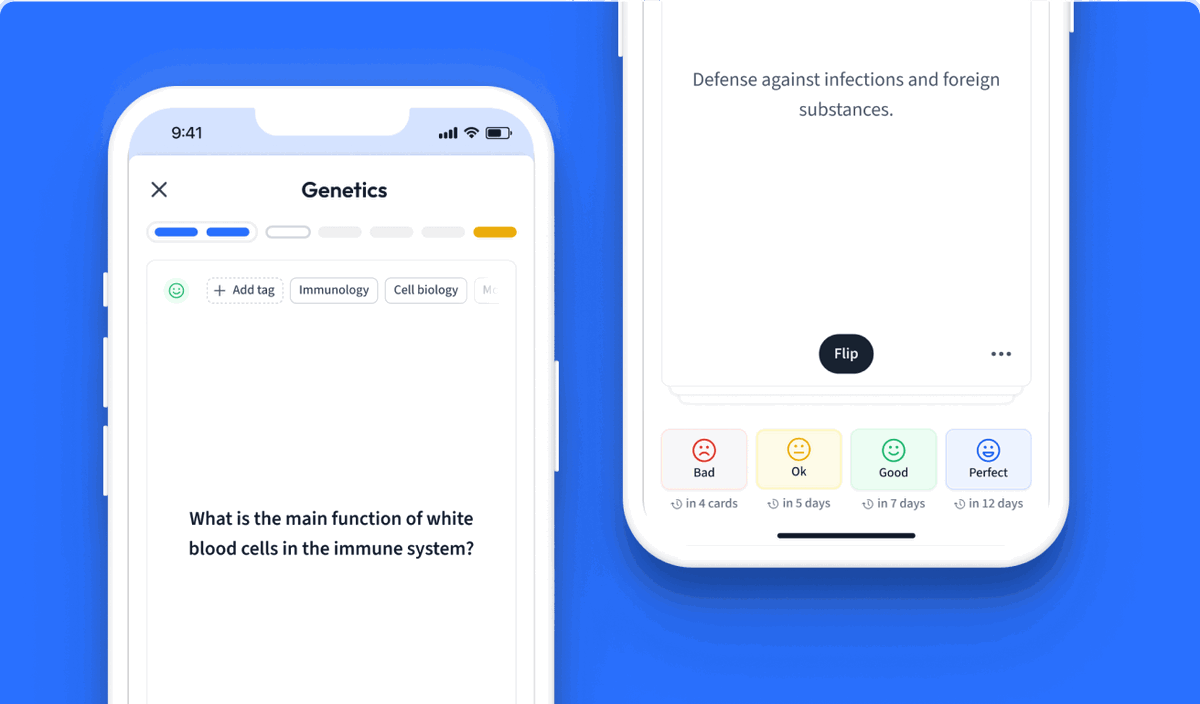
Learn with 11 Analytical Techniques flashcards in the free StudySmarter app
We have 14,000 flashcards about Dynamic Landscapes.
Already have an account? Log in
Frequently Asked Questions about Analytical Techniques
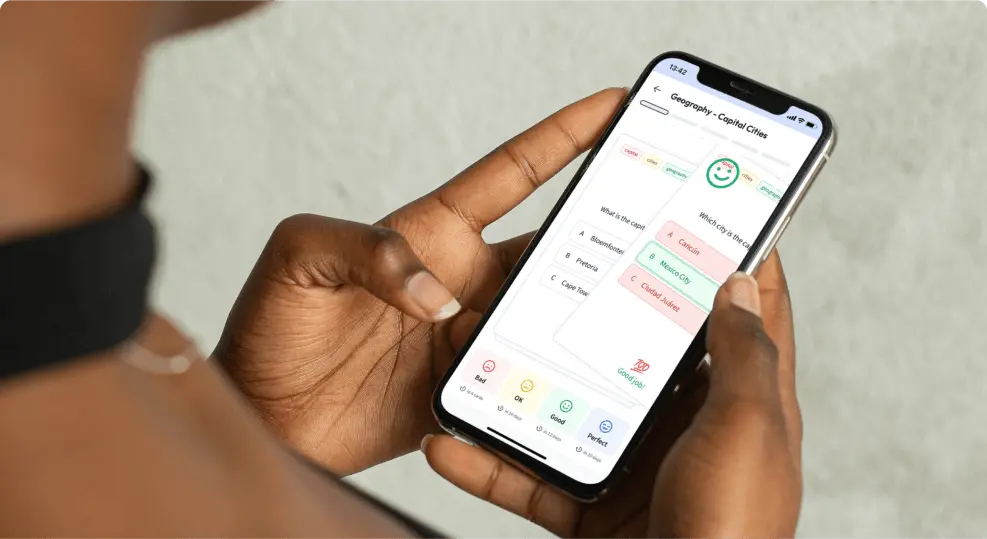
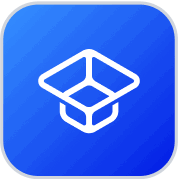
About StudySmarter
StudySmarter is a globally recognized educational technology company, offering a holistic learning platform designed for students of all ages and educational levels. Our platform provides learning support for a wide range of subjects, including STEM, Social Sciences, and Languages and also helps students to successfully master various tests and exams worldwide, such as GCSE, A Level, SAT, ACT, Abitur, and more. We offer an extensive library of learning materials, including interactive flashcards, comprehensive textbook solutions, and detailed explanations. The cutting-edge technology and tools we provide help students create their own learning materials. StudySmarter’s content is not only expert-verified but also regularly updated to ensure accuracy and relevance.
Learn more