The stages of a research experiment
In order to see how we can go about drawing conclusions from our data, we need to be keeping a few things in mind throughout the process. First, we need to think about what we expect to see from the data. Our knowledge of the subject matter and the data itself should help us decide how to go about our analysis. After this, we will be in a good position to say whether our analysis either confirms or denies our original expectations about the data.
The hypothesis
The very first thing a researcher (that's you!) does - before the experiment and certainly before analyzing the experimental data - is come up with a hypothesis. This is a very specific prediction about what we might expect the data to show. It is important to set up the hypothesis before we analyze the data since this will determine the ways in which we look at the data.
Often, the hypothesis will be defined for you in the question, but it is still useful to keep it in mind throughout the analysis and conclusion.
Hypothesis: a specific prediction about the outcome from an experiment that is used as the starting point for research. It is usually either proven or disproven by the end of an experiment.
Say we wanted to observe the effect of sunny weather on the revenue of a lemonade stand. We might define our hypothesis to be: the revenue of the lemonade stand is higher when the sun is out.
Using common sense, people are more likely to want to buy cold drinks on sunny days, and this will probably affect the revenue. Without any more information about the situation, there is nothing else we can go by.
The experiment and analysis
As per the example, say we collected the following data:
Revenue, r (dollars) | Frequency when sunny | Frequency when not sunny |
2 | 52 | |
3 | 49 | |
17 | 29 | |
29 | 27 | |
57 | 17 | |
62 | 8 | |
30 | 0 |
Since the data is grouped, we can plot each of the sets of data using a histogram.
Histogram 1, StudySmarter Originals

From the graphs, we can see that the mode class for the sunny data is the class, whereas for the non-sunny weather the mode class is .
We can also find the mean as an additional measure of central tendency. Recall that to find the mean from grouped data, we need to use the midpoints of each class.
Revenue, x (dollars) | Frequency when sunny, | Frequency when not sunny, | Class midpoint, m | m | m |
2 | 52 | 7.5 | 15 | 390 | |
3 | 49 | 22.5 | 67.5 | 1,102.5 | |
17 | 29 | 35 | 595 | 1,015 | |
29 | 27 | 45 | 1,305 | 1,215 | |
57 | 17 | 55 | 3,135 | 935 | |
62 | 8 | 65 | 4,030 | 520 | |
30 | 0 | 80 | 2,400 | 0 |
Now we can find the mean for the 'sunny days' revenue:
to 2 d.p.
And now find the mean for the 'non-sunny days' revenue:
to 2 d.p.
The conclusion
So, we have collected and analyzed the data. All that is left to do is to compare our statistics with the hypothesis we made beforehand.
Recall: the revenue of the lemonade stand is higher when the sun is out.
Now we compare our original hypothesis with the statistics we have found. We can see that the average amount of revenue on a sunny day is $59.95, while the average revenue on a day that is not sunny is $18.24. Since the sunny-day revenue is so much higher than otherwise, we can conclude that the data we have collected supports our hypothesis and that, according to the data, the revenue of the lemonade stand is higher when the sun is out.
This process - drawing a conclusion about a population based on results collected from a sample - is called statistical inference.
But beware! It is important to be careful of the language we use in our hypothesis. Take for example the following statement: "sunny weather makes people more likely to buy lemonade". While this may be true, we don't know enough from the data itself to confirm that it was the weather specifically that inspired more people to buy lemonade. Instead, it could have been the case that the weather increased the number of potential customers in the vicinity of the lemonade stand.
We can also use other statistics to add to our conclusion. We can see that the range of the 'sunny day' revenues is 90 whereas the range of the 'not sunny days' is 70. Therefore we can also add to our conclusion that while there is on average a greater amount of revenue on sunny days, there is also a greater range in the revenue.
Your conclusion must always refer specifically to what the data shows us!
Drawing Conclusions from Examples - Key takeaways
- The hypothesis is a specific prediction about the outcome of an experiment that is used as the starting point for research. It is usually either proven or disproven by the end of an experiment
- To draw conclusions, we first must collect the relevant data and perform statistical analyses such as creating visualizations of the data (e.g. a histogram) and finding relevant statistics (e.g. the mean)
- In the conclusion, compare the statistical analysis with your original hypothesis
- The process of drawing a conclusion about a population based on results collected from a sample is called statistical inference
- Be careful in your conclusion to only refer to what the statistics specifically tell us about the data
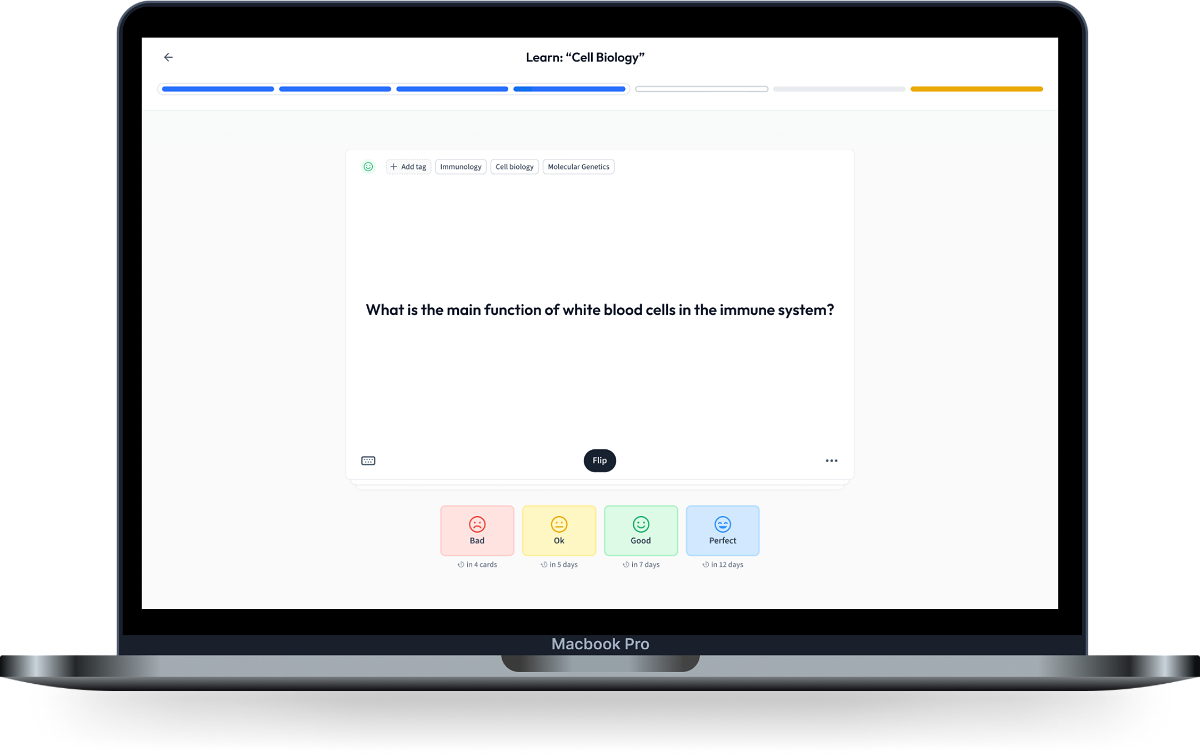
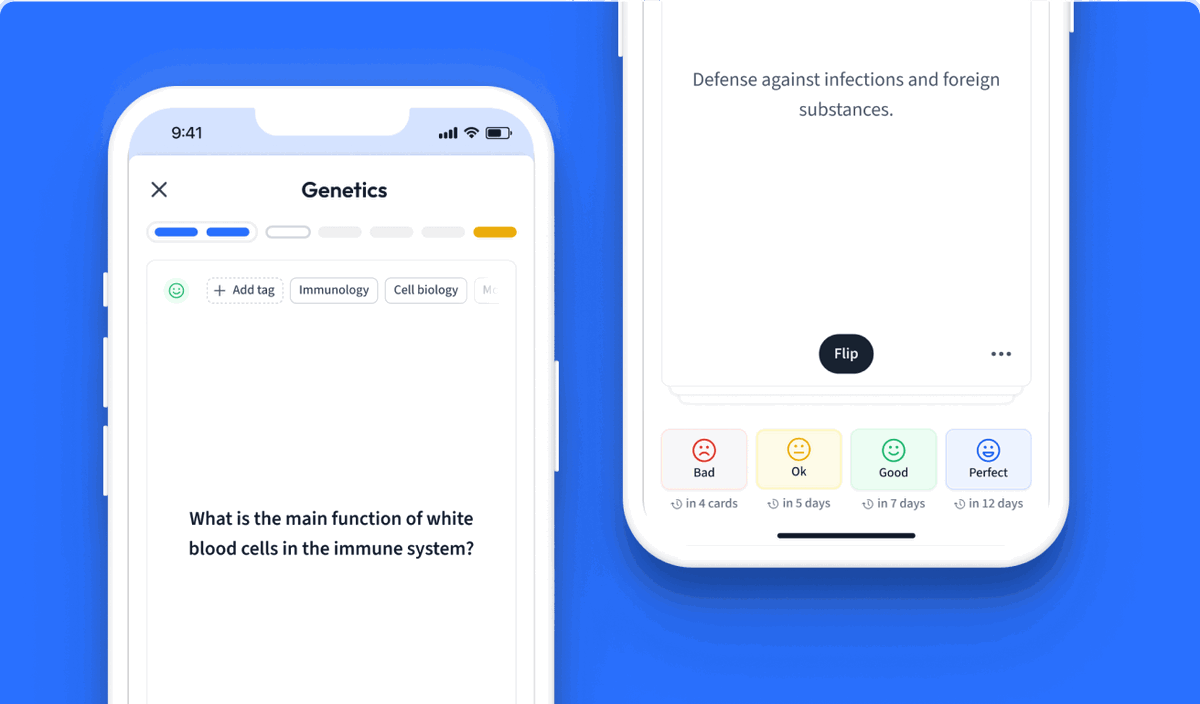
Learn with 27 Drawing Conclusions from Examples flashcards in the free StudySmarter app
We have 14,000 flashcards about Dynamic Landscapes.
Already have an account? Log in
Frequently Asked Questions about Drawing Conclusions from Examples
If the average time spent doing math homework is higher than the average time doing biology homework, we draw the following conclusion: 'on average, students spend more time doing math homework than biology homework'.
How do you draw conclusions from examples?
In the conclusion of an experiment, you should compare your original hypothesis with statistics you have derived from the data.
Drawing a conclusion means using statistics to make a statement about the thing you have measured in the experiment. The process of drawing a conclusion about a population based on results collected from a sample is called statistical inference.
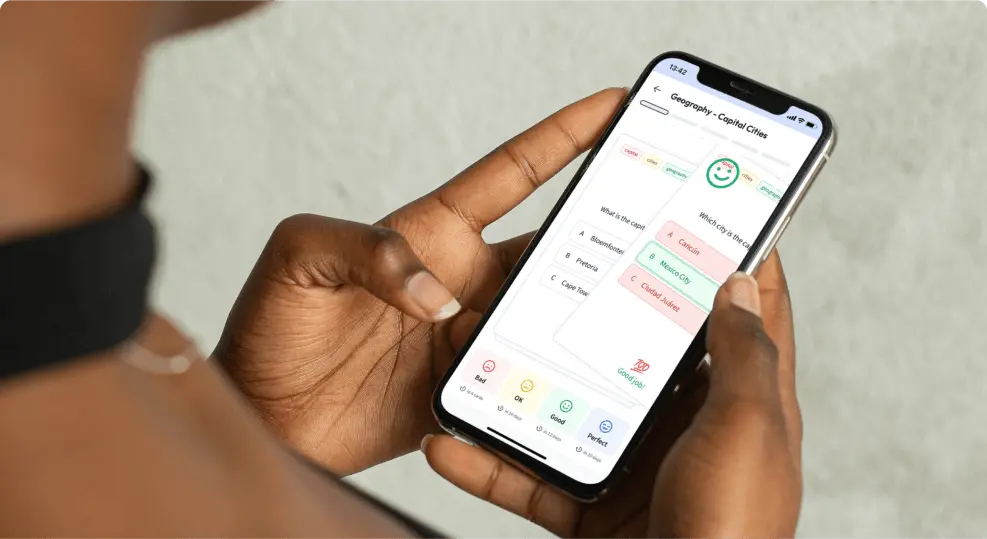
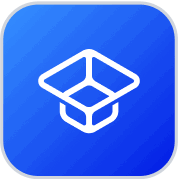
About StudySmarter
StudySmarter is a globally recognized educational technology company, offering a holistic learning platform designed for students of all ages and educational levels. Our platform provides learning support for a wide range of subjects, including STEM, Social Sciences, and Languages and also helps students to successfully master various tests and exams worldwide, such as GCSE, A Level, SAT, ACT, Abitur, and more. We offer an extensive library of learning materials, including interactive flashcards, comprehensive textbook solutions, and detailed explanations. The cutting-edge technology and tools we provide help students create their own learning materials. StudySmarter’s content is not only expert-verified but also regularly updated to ensure accuracy and relevance.
Learn more